Chapter 2 – Visual Analytics
This chapter describes some application areas for visual analytics and puts the size of the problem into context, and elaborates on the definition of visual analytics. The interdisciplinary nature of this area is demonstrated by considering the scientific fields that are an integral part of visual analytics.
Definition
Visual analytics is not easy to define, due to its multi-disciplinary nature involving multiple processes and the wide variety of application areas. An early definition was “The science of analytical reasoning facilitated by interactive human-machine interfaces” [Wong and Thomas 2004]. However, based on current practice, a more specific definition would be: “Visual analytics combines automated analysis techniques with interactive visualisations for an effective understanding, reasoning and decision making on the basis of very large and complex datasets”.
Application of visual analytics
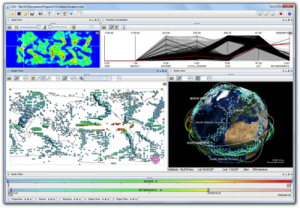
Visual support for the simulation of climate models provided by CGV (Coordinated Graph Visualization), a highly interactive graph visualisation system
Visual analytics is essential in application areas where large information spaces have to be processed and analysed. Major application fields are physics, astronomy, climate monitoring, emergency management, security, biology, medicine and commerce. For example, monitoring climate and weather is a domain which involves huge amounts of data collected by ground or water based sensors throughout the world and from satellites. A visual approach can help to interpret the massive amounts of data and to gain insight into the dependencies of climate factors and climate change scenarios that would otherwise not be easily identified. An example of visualising hurricane paths to aid the insurance industry can be found here.
The visual analytics process
Combines automatic and visual analysis methods with a tight coupling through human interaction in order to gain knowledge from data. Heterogeneous data sources need to be integrated before visual or automatic analysis methods can be applied (e.g. data cleaning, normalisation, grouping, integration). Analyst applies visual or automatic analysis methods. Automated analysis uses data mining techniques to generate models of the original data that can be visualised for evaluation and refinement. Alternating between visual and automatic methods is characteristic for the visual analytics process. Misleading results in an intermediate step can thus be discovered at an early stage, leading to better results and a higher confidence. Findings in the visualisations can be used to steer model building in the automatic analysis.
Building blocks of visual analytics research
Visual analytics integrates science and technology from many disciplines. Visualisation is at the heart of the system, not only is it the means to communicate data values or the results of some analysis, but it is also increasingly being used to monitor processes in other disciplines, such as data management and data mining. Infrastructure and evaluation are important to all the disciplines. Infrastructure links together all the processes, functions and services required by visual analytic applications so they work in harmony. Evaluation assesses the effectiveness, efficiency and user acceptance of the methods, models and theories in a standard way, so they can be compared and problems can be identified.
Did you know:
- On a worldwide scale, per day there are upwards of 210 billion emails, 4 billion SMS messages and 90 million tweets.
- The Europe Media Monitor collects news documents from 2,500 news sources: media portals, government websites, and news agencies and processes 80,000-100,000 articles per day in 43 languages.
Download
chapter 2 (1.5MB) [Note that the images are low res. to reduce the file size]
0 Comments
You can be the first one to leave a comment.